Measuring translation difficulty:
Theoretical and methodological considerations
SANJUN SUN
E-mail: sunsanjun@gmail.com Across Languages and Cultures 16 (1), pp. 29–54 (2015)Abstract : Accurate assessment of a text’s level of translation difficulty is critical for translator training, accreditation and research. Traditionally, people rely on their general impression to gauge a text’s translation difficulty level. If the evaluation process is to be more effective and the results more objective, an instrument needs to be developed. Then two basic research questions must be answered: what to measure and how to measure it. The potential sources of translation difficulty include translation factors (i.e., text difficulty and translation-specific difficulty) and translator factors. Accordingly, to measure translation difficulty, we need to measure text difficulty, identify translation-specific difficulty, and assess translation difficulty (i.e., mental workload) for the translator. Readability formulas are often used to measure text difficulty. The means for identifying translationspecific difficulty include grading translations, analyzing verbal protocols, and recording and analyzing translation behavior. For measuring mental workload, we can adopt subjective measures (e.g., a multidimensional rating scale), performance measures, or physiological measures. This article intends to provide a theoretical and methodological overview of translation difficulty and serve as a foundation for this line of inquiry.
Keywords : translation difficulty, mental workload, cognitive load, readability, competence, measurement
1.INTRODUCTION
Knowing the difficulty level of a translation assignment is important in translation pedagogy, accreditation and research. Traditionally, people rely on their holistic intuitions to gain an idea of the level of a text’s translation difficulty. Although experts’ intuitions (especially an expert panel’s judgment) is “reasonably reliable” (Campbell and Hale 1999), we still need instruments or established procedures to make the evaluation process more effective and the results more objective.
In the field of foreign language teaching, research has demonstrated that providing an appropriate level of challenge (i.e., instructional level) improves academic outcomes (see Burns 2004). For example, an optimal instructional
level for reading has been suggested to contain 93% to 97% known words, with 3% to 7% new or unknown words (Gickling and Rosenfield 1995). The implication for translator education is that translation tasks should be sequenced in an order of increasing complexity for students, and the difficulty level of source texts should be appropriate for them. Hence, there is a need for properly leveled passages for translation exercises in translation pedagogy.
Assessing the translation difficulty level of testing materials is a must for translation accreditation bodies. Unfortunately, because of lack of research in this field, their assessments are not always as effective as intended. For instance, this author found that, when studying the American Translators Association (ATA)’s 2006 English–Chinese examinations, among the three test passages A (general), B (in the domain of medicine), and C (in the domain of business), passage C was the most difficult one to comprehend according to the readability test results, and the passing rate for passage C was the lowest among the passages. As the ATA intended the passages to be equally difficult, this finding makes one doubt that their workgroup method for evaluating the translation difficulty level of those texts in 2006 was effective.
In translation research, translation difficulty deserves more attention. For instance, in process-oriented research, researchers have no standards to refer to when they choose test passages, and the texts used are diverse in terms of text type, length and, possibly, difficulty (see Krings 2001:74). This makes it hard for one to evaluate the comparability of experimental results between these studies. For example, the use of different translation strategies in terms of type and frequency might vary depending on the translation difficulty level of the texts. Dragsted (2004) found in her empirical study that professional translators would adopt a more novice-like behavior during translation of a difficult text than during the translation of an easy text. Thus, translation difficulty is an important variable in translation process research.
Although the expression ‘translation difficulty’ has been mentioned frequently in translation literature (e.g., Nord 2005), few researchers have attempted to measure it. Campbell and Hale (e.g. 1999, 2002), Jensen (2009), and Liu and Chiu (2009) are among the few researchers who have done some exploratory empirical work in this direction. Campbell and Hale (2003) argue that it is possible to compile an inventory of universal sources of translation difficulty in source texts as well as inventories for specific language pairs. By contrast, in the area of research on reading, researchers have been working on readability formulas for measuring text readability since the 1920s.
This article intends to provide a theoretical and methodological overview of translation difficulty and serve as a foundation for this line of inquiry. In order to measure translation difficulty, we have to specify what to measure and how to measure it. Figure 1 is an outline of the structure of this field.
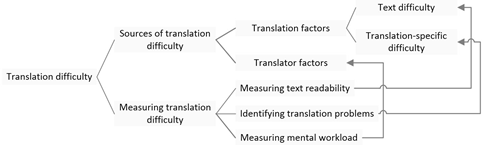
Figure 1
Measuring translation difficulty
To begin, we need to know what translation difficulty is.
2.WHAT IS TRANSLATION DIFFICULTY?
From the cognitive perspective, difficulty refers to the amount of cognitive effort required to solve a problem. Difficult tasks require more processing effort than easy ones. Translation difficulty can be viewed as the extent to which cognitive resources are consumed by a translation task for a translator to meet objective and subjective performance criteria. Difficulty is similar to complexity, but the two are not the same. According to Dahl (2004:39), difficulty is a notion that primarily applies to tasks, and is always relative to person, hence the term ‘task difficulty’; complexity as an information-theoretic notion is more objective in the sense of being independent of use and can be computed mathematically. Complexity is an important component of difficulty.
Mental workload and cognitive load are similar to difficulty, and yet they have different theoretical backgrounds. The two will be discussed in the following paragraphs.
Mental workload has been an important concept in the field of human factors and ergonomics (see, e.g., Moray 1979), which is concerned with the design and evaluation of tasks, products, and systems in order to make them compatible with the needs, abilities, and limitations of people (Karwowski 2006:i). To date, there is still no universally accepted definition of mental workload. An oft-quoted one is by Gopher and Donchin (1986): mental workload refers to “the difference between the capacities of the information processing system that are required for task performance to satisfy performance expectations and the capacity available at any given time” (p. 41).
It is generally accepted that mental workload is a multidimensional construct (e.g., Hart and Staveland 1988). A cohesive and multidimensional model
incorporating factors affecting mental workload was proposed by Meshkati (1988). This model consists of two major sections – Causal Factors and Effect Factors – each with two primary component groups. The Causal Factors are 1) Task and Environmental Variables, which include task criticality, intrinsic taskrelated variables (including amount of information); time pressure, task structure and its rigidity (e.g., decoding requirements, decision making vs. problem solving), task novelty (to the operator); task rate/frequency; equipment used; and type of reward system, and 2) Operators’ Characteristics and Moderating Variables, which include cognitive capabilities of the individual (e.g., intellect), motivational states and personal utility system, past experience and training, etc. The Effect Factors include 1) task difficulty, response and performance, and 2) mental workload measures. These factors interact with each other.
The term ‘cognitive load’ has been used in psychology since the 1960s (e.g., Bradshaw 1968). It can be generally defined as “the demand for working memory resources required for achieving goals of specific cognitive activities in certain situations” (Kalyuga 2009:35), although scholars in different fields have different ideas about this term. For instance, researchers in the field of human factors and ergonomics (e.g., Sammer 2006) take cognitive load (as well as informational load, attentional load, and emotional load) as a subconcept of workload.
Cognitive load theory (CLT) was first advanced in 1988 by Sweller. It has attracted a group of researchers in educational psychology and applied learning sciences, and has been expanded significantly ever since (see, e.g., Sweller et al. 2011). This line of research originated in studies of human learning during performance of problem-solving tasks. Its objective is to predict learning outcomes by taking into consideration the capabilities and limitations of human cognition (Plass et al. 2010:1). According to CLT, the magnitude of cognitive load is determined by the degree of interactivity between three factors: 1) the individual learner’s expertise level (especially prior knowledge and effective working memory size); 2) the instructional material’s complexity; 3) the specific task.
Despite their differences in research scope and purpose, task difficulty, mental workload, and cognitive load are more similar than different. They all take into consideration the information processing demands that a certain task imposes on an individual and the limits of working memory. Compared with the other two constructs, mental workload is far more established in terms of breadth, impact and number of researchers in the field. Thus, mental workload theories can provide the major theoretical basis for translation difficulty research. Yet, we will use “translation difficulty” rather than “translation mental workload” or “translation cognitive load” as the cover term for this concept for two reasons: 1) the term “difficulty” is more commonly used than “mental workload” or “cognitive load” in Translation Studies (e.g., Nord 2005) and related
fields like second language acquisition (e.g., Robinson 2011); 2) “mental workload” and “cognitive load” as terms seem to lay emphasis on the operator’s cognitive processes, while “translation difficulty” stresses the property of a task, which is more in line with the ultimate object of our measurement.
3.SOURCES OF TRANSLATION DIFFICULTY
Based on the discussion in the preceding section (especially the mental workload model proposed by Meshkati), sources of translation difficulty can be divided into two groups: task (i.e., translation) factors and translator factors. They are the factors that need to be measured.
3.1 Translation Factors
The translation process consists of reading (also called by different scholars “comprehension”, “decoding”, “analysis”) and reverbalization (also called “writing”, “encoding”, “recoding”, “rendering”, “reformulation”, “retextualization”, “synthesis”) (e.g., Wilss 1982). The translator reads the source text in the first phase and reverbalizes the meaning of the source text in the target language in the second phase. Reading comprehension is a broad research field (for an overview, see, e.g., Kamil et al. 2011), and the subfield that is particularly pertinent to translation difficulty research is text difficulty. Factors that may cause difficulty in the second phase will be grouped under the heading “translationspecific difficulty” rather than “reverbalization” (or other terms) in order to facilitate the connection with existing literature on translation. Between these two groups of factors there are unavoidable overlaps.
3.1.1 Text difficulty
Text difficulty and readability are a very important topic in reading research. The RAND Reading Study Group (2002:25), a 14-member panel funded by the United States Department of Education’s Office of Educational Research and Improvement, propose the following categories and dimensions that vary among texts and create varying challenges for readers:
- discourse genre, such as narration, description, exposition, and persuasion;
- discourse structure, including rhetorical composition and coherence;
- media forms, such as textbooks, multimedia, advertisements, and the Internet;
- Sentence difficulty, including vocabulary, syntax, and the propositional text base;
- content, such as age-appropriate selection of subject matter;
- texts with varying degrees of engagement for particular classes of readers.
Most of these categories are also mentioned by Gray and Leary in their book What Makes a Book Readable (1935). These categories will be discussed in more detail in the following paragraphs.
3.1.1.1 Lexical and syntactic complexity
Gray and Leary tested the reading ability of 1,690 adults and found through statistical analysis that the best estimate of a text’s difficulty involved the use of eight elements: number of different hard words, number of easy words, percentage of monosyllables, number of personal pronouns, average sentence length in words, percentage of different words, number of prepositional phrases, and percentage of simple sentences (p. 16). These are all structural elements in the style group, as they “lend themselves most readily to quantitative enumeration and statistical treatment” (p. 7).
3.1.1.2 Content and subject matter
In terms of content and subject matter, it is commonly believed that abstract texts (e.g., philosophical texts) will be harder to understand than concrete texts describing real objects, events or activities (e.g., stories), and texts on everyday topics are likely to be easier to process than those that are not (Alderson 2000:62). This can be explained by schema theory, which has been an important theory in reading comprehension since the 1980s.
Usó-Juan (2006) tested 380 native Spanish-speaking undergraduates who exhibited a wide range of proficiency in English as a foreign language and knowledge of the academic topics being tested, and found that English proficiency accounted for a range varying between 58 and 68% of EAP (i.e., English for academic purposes) reading, whereas discipline-related knowledge accounted for a range varying between 21 and 31%. This illustrates the importance of prior knowledge of a topic.
3.1.1.3 Text type
The first two categories and dimensions by RAND mentioned above, i.e., discourse genre and discourse structure, can actually be discussed within the framework of text typology. The concepts ‘genre’ ‘register’ ‘style’ ‘text type’ ‘domain’ are not easily distinguished from each other. Researchers from different backgrounds hold different opinions (for overviews, see Lee 2001, Moessner 2001). In this study, ‘text type’ will be used as an overarching term.
In the field of translation studies, researchers have proposed different text classification methods (for an overview see e.g., Trosborg 1997). For instance, Snell-Hornby (1988) proposes a text categorization method based on the gestalt principle and the concept of prototypology. Her integrated approach diagram includes many levels. On the horizontal plane the diagram represents a spectrum from literary translation to general language translation and to special language translation. As the degree of variation in text difficulty within each text type is large, it is not possible for us to make such assertions as “modern literature is more difficult to translate than legal texts”. As a result, such subjective function-based text classifications are not particularly useful for discussions about text difficulty or translation difficulty.
By analyzing the co-occurrence distributions of 67 linguistic features in 481 spoken and written texts of contemporary British English using factor analysis, Biber (1988, 1989) develops a typology of texts in English with respect to a five-dimensional model of variation. The five dimensions are: 1) involved vs. informational production; 2) narrative vs. non-narrative concerns; 3) elaborated vs. situation-dependent reference; 4) overt expression of persuasion; abstract vs. non-abstract style. Each dimension consists of a set of lexical and syntactic features that co-occur frequently in texts. The relevance of Biber’s text typology to translation difficulty research is that the linguistic features he used or dimensions (i.e., clusters of lexical and syntactic features) might cause difficulty in reading or translation. In Translation Studies, Hatim and Mason (1997:24) proposed a model of analyzing texts, which includes six textual features (i.e., cohesion, coherence, situationality, intentionality, intertextuality, and informativity) on a static-dynamic continuum. In the field of reading research, there have been some conclusions like “expository texts are harder to process than narrative texts”, and this might be “because of the greater variety of relationships among text units, possibly due to greater variety of content” (Alderson 2000:64). All these call for empirical studies.
3.1.2 Translation-specific difficulty
In the field of translation, Nord (1991/2005) divides into four categories translation problems that every translator has to solve irrespective of their level of competence: 1) text-specific translation problems (e.g., a play on words), 2) pragmatic translation problems (e.g., the recipient orientation of a text), 3) cultural translation problems (e.g., text-type conventions), and 4) linguistic translation problems (e.g., the translation of the English gerund into German). Shreve et al. (2004) list the following factors: 1) textual or discourse variance, 2) textual degradation including fragmentary texts and illegible texts, 3) linguistic ‘distance’ between the source text and the target text, 4) cultural ‘distance’ between the source culture and the target culture, 5) lexical complexity, 6) syntactic complexity, 7) conceptual, topical or propositional complexity. The above delineations are all theoretical explorations.
Campbell and Hale are among the few researchers who have looked into translation difficulty empirically. They (1999) have identified several areas of difficulty in lexis and grammar, that is, words low in propositional content, complex noun phrases, abstractness, official terms, and passive verbs.
We can see that there is considerable overlap between these lists and it seems that the three major aspects causing reading difficulty mentioned in the previous section, that is, lexical and syntactic complexity, content and subject matter, and text type have also been covered.
What differentiates aspects of text difficulty from aspects of translationspecific difficulty can be narrowed down to one concept: equivalence. Equivalence is an important concept in translation theory (for an overview, see Kenny 2009). It is “a relation of ‘equal value’ between a source-text segment and a target-text segment”, and “can be established on any linguistic level, from form to function” (Pym 2010:7). In her classic translation textbook In Other Words, Baker (1992/2011) discusses equivalence at a series of levels: lexical (word level and above-word level), grammatical (e.g., number, gender, person, tense and aspect, and voice), textual (e.g., cohesion), and pragmatic (e.g., coherence and implicature). Non-equivalence, one-to-several equivalence, and one-to-part equivalence situations can create difficulty for translators, especially for novice translators. The actual difficulty level of a translation task involves translator competence, which will be dealt with in the next section.
3.2 Translator Factors
As mentioned above, the operator factors mainly include the individual’s cognitive capabilities, past experience and training (i.e., prior knowledge). In the recent
two decades, translation process researchers have been looking into these factors empirically (see Jääskeläinen 2002) and these translator factors together are usually called “translation competence”.
Competence refers to personal qualities, skills and abilities, and exists in different degrees (Englund Dimitrova 2005:16). So far, many categories of translation subcompetences have been proposed. For instance, Wilss (1976) suggests three subcompetences: 1) a receptive competence in the source language, 2) a productive competence in the target language, and 3) a supercompetence for transferring messages between linguistic and textual systems of the source culture and those of the target culture. Neubert (2000) proposes the following classification: 1) language competence, 2) textual competence, 3) subject competence, 4) cultural competence, and 5) transfer competence.
The translation competence model proposed by the PACTE group in 2003 is influential. Based on empirical (mostly qualitative) studies, this model comprises five subcompetences: 1) bilingual subcompetence, 2) extra-linguistic subcompetence, 3) knowledge about translation subcompetence, 4) instrumental subcompetence, and 5) strategic subcompetence. We can see that, except for transfer competence, the categories mentioned by Wilss and by Neubert are all included in the PACTE model. The PACTE group argues that the transfer subcompetence is not just one subcompetence because “[a]ll bilinguals possess a rudimentary transfer ability” (p. 57). They have replaced it with the strategic subcompetence, which refers to procedural knowledge for identifying translation problems and applying procedures to solve them. We can see that the strategic subcompetence is basically problem-solving ability, and it is widely accepted that problem solving is an aspect of human intelligence (e.g., Lohman and Lakin 2011). In other words, these subcompetences are further categorizations of the individual’s cognitive capabilities and prior knowledge mentioned at the beginning of this section.
Naturally, different translation tasks require the use of certain subcompetences of translation more than others. For instance, if a source text contains a large number of terms unknown to the translator, then instrumental subcompetence (e.g., knowledge related to the use of the Internet and dictionaries) will be critical. On these subcompetences translators differ; each translator has a unique combination of these subcompetences at various levels.
Since the mid-1980s, translation process researchers (e.g., Lörscher 1986) have been comparing translators of different competence levels and exploring aspects of translation competence. For lack of a theoretical framework in this field, these studies differ widely in their focus, scope and applicability to specific purposes (Fraser 1996). Later, expertise theories were formally introduced into translation studies by Shreve (2002:168), who makes an appeal to “leverage
the expertise studies research to generate hypotheses or research questions for translation scholars to address”.
It is necessary to relate the findings in the expertise research field to the results of translation process studies. This may shed light on the characteristics of translation competence and its acquisition process. For instance, Chi (2006) summarizes seven key characteristics enabling experts’ successful performance, e.g., experts are more successful at choosing the appropriate strategies to use than novices. It seems that we can find supporting evidence for most of these characteristics in the field of translation. One exception concerns the following characteristic: experts excel in generating the best solution and can do this faster and more accurately than non-experts (p. 23). In Tirkkonen-Condit’s twoparticipant experiment (1987) involving one first-year student and one fifth-year student of translation, the mature student identified more problems but spent less time on the process. By contrast, Jääskeläinen (1999) found that the four non-professional translators spent less time on the task than the four professionals. Clearly, this question needs more research.
4. MEASURING TRANSLATION DIFFICULTY
Section 3 dealt with potential sources of translation difficulty, which include translation factors (i.e., text difficulty and translation-specific difficulty) and translator factors. Accordingly, in order to measure translation difficulty, we need to measure text difficulty, identify translation-specific difficulty (i.e., translation problems in a task), and assess translation difficulty (i.e., mental workload) for the translator.
4.1 Measuring Text Readability
To measure text difficulty, reading researchers have tended to focus on developing readability formulas since the early 1920s. A readability formula is an equation which combines the statistically measurable text features that best predict text difficulty, such as average sentence length in words or in syllables, average word length in characters, and percentage of difficult words (i.e., words with more than two syllables, or words not on a particular wordlist). Until the 1980s, more than 200 readability formulas had been published (Klare 1984). Among them, the Flesch Reading Ease Formula, the Dale–Chall Formula, Gunning Fog Index, the SMOG Formula, the Flesch–Kincaid Readability test, and the Fry Readability Formula are the most popular and influential (DuBay 2004). These formulas use one to three factors with a view to easy manual application.
Among these factors, vocabulary difficulty (or semantic factors) and sentence length (or syntactic factors) are the strongest indexes of readability (Chall and Dale 1995). The following is the Flesch Reading Ease Formula.

The resulting score ranges from 0 to 100; the lower the score, the more difficult to read the material. A score of 0 to 30 indicates the material is ‘very difficult’ (graduate school level); 60 to 70, ‘standard’; 90 to 100, ‘very easy’ (4th grade level) (Flesch 1948:230).
According to Klare (1984, from Anagnostou and Weir 2007:6), the steps to produce a readability formula are:
- Choose (or develop) a large set of criterion-text passages of varied content and measured difficulty (typically comprehension) values.
- Identify a number of objectively stated language factors.
- Count the occurrences of the language factors in the criterion passages and correlate them with the difficulty values of the criterion passages.
- Select the factors with the highest correlations as potential indexes of readability.
- Combine the indexes, using multiple regression techniques, into a formula.
- [Optional] Cross-validate on a set of new criterion passages.
These steps can be adopted in studying translation difficulty and will be briefly explained below.
4.1.1 Obtaining the reading difficulty value of a passage
The method for deriving the reading difficulty values of test passages used most frequently by readability formula developers is probably the cloze procedure developed by Taylor (1953), in which test developers delete every fifth word from a passage and readers are asked to accurately guess the word that belongs in each blank. The cloze score is the number of exact word replacements divided by the number of deleted words, expressed as a percentage. A passage that is assigned a difficulty value is called a criterion passage.
Subjective judgments are also used to measure the reading difficulty of a passage. Carver (1975–1976) developed a technique called the Rauding Scale that involves using the subjective ratings of qualified experts for estimating the
difficulty of reading material. Chall et al. (1996) present a method that relies on overall impression rather than on an analysis of text features for the qualitative assessment of text difficulty. The method is based on matching a text to exemplars that have been scaled for comprehension difficulty in terms of vocabulary difficulty, sentence length and complexity, conceptual difficulty, idea density and difficulty. It is a pairwise comparison, in which pairs of texts are compared to decide which of the two has a greater amount of some quantitative property.
4.1.2 Identifying the language factors and counting their occurrences
There are many language factors whose occurrences in a passage can be objectively counted. Gray and Leary (1935) identified 289 factors that may be related to a book’s readability, and 64 of them are countable. Biber (1989:7) analyzed the co-occurrence distributions of 67 linguistic features, which fall into 16 major grammatical categories, such as tense and aspect markers, pronouns and proverbs, passives, and lexical classes. These features can be identified automatically with the help of computer programs.
There are many text analysis tools currently available for analyzing various aspects of a text, e.g., syntax, semantics, propositions, text cohesion and coherence, and text type (see Castello 2008; Graesser et al. 2011). If some language features cannot be identified with computers, manual annotation of these features can facilitate automatic analysis.
4.1.3 Combining the indexes into a formula
This involves a statistical technique called multiple regression analysis, which is one of the most widely used statistical techniques in the social sciences. Multiple regression is for investigating the joint effect of one or more predictor variables (e.g., average sentence length, average word length) on a response variable (e.g., readability). It can determine which predictor variables are important predictors of the response variable, and predict the value of the response variable from the predictor variables (see e.g., Ott and Longnecker 2010).
Let us take Bonnuth’s 1964 study (an example from Chall and Dale 1995). He tested and correlated 47 readability variables with his criterion passages and found that the linguistic factors other than traditional measures of readability (e.g., sentence length, word length) added little to the overall predictions of reading difficulty.
It should be noted that multiple regression analysis can reveal how variables are related to each other, and whether some variables can predict a response
variable, but cannot prove causal relations among variables (Urdan 2010:156). That is, the readability formula does not necessarily imply causal connections between the chosen predictor variables (e.g., vocabulary difficulty, sentence length) and the response variable (i.e., readability). Reading difficulty often comes from the ideas rather than the words or sentences. However, difficult passages that express difficult, abstract ideas tend to contain hard words, while easy passages dealing with familiar, concrete ideas tend to use familiar words (Rayner and Pollatsek 1989:319). In addition, according to Zipf’s law (Zipf 1935), word frequency and word length are inversely related, i.e., short words occur with high frequency while longer words occur with lower frequency as a result of a biological principle of least effort. These might explain why readability formulas work.
Over the decades readability formulas have been proved to be valid and fairly reliable (e.g., Fry 1989, Klare 1974–1975), and have been adopted by publishers, schools, and software producers (e.g., the Flesch Reading Ease Formula and Flesch–Kincaid Readability Formula are used by Microsoft Word).
However, the readability formulas mentioned so far are all for English native speakers (especially people in the USA). In the professional world, translators are usually expected to work from a foreign language into the mother tongue (Lonsdale 2009), and English has been the most translated language (Sin 2011). That is, most translators translate from English into their mother tongue. The pertinent question is whether the mainstream readability formulas work for EFL (English as a Foreign Language) or ESL (English as a Second Language) students. More research is needed in this regard.
4.2. Identifying Translation Problems
There are many ways to locate translation difficulty ‘points’ in a source text for a translator. The three methods common in translation studies are translation quality assessment (or more specifically, grading translations), analyzing verbal protocols, and recording and analyzing translation behavior, which will be detailed in the following sections. But first, it is necessary to review the methods used by Campbell and Hale and by Jensen (2009), who are among the few researchers who have investigated translation difficulty empirically.
The way Hale and Campbell (2002) assessed the difficulty of a source text was to count the number of different renditions in a group of translators translating that text. Their rationale was that “the different renditions represent the options available to the group of subjects, and that each subject is faced with making a selection from those options”; “where there are numerous options, each subject exerts relatively large cognitive effort in making a selection; where there
are few options, each subject exerts relatively small cognitive effort” (p. 15). Hale and Campbell seemed to assume that the subjects were faced with the same number of options in translation. This contradicts Pym’s (2003) view of translation competence. Pym identifies two components of translation competence, namely, 1) the ability to generate a series of more than one viable target text for a pertinent source text; 2) the ability to select only one viable target text from this series, quickly and with justified confidence (p. 489). In other words, poor translators generate fewer translation equivalents than do good translators, and are faced with making a selection from fewer options (or even one or none). In addition, if a proper name has only one equivalent in the target language, and it takes the translators a lot of time to find that equivalent, is this easy or difficult? According to Hale and Campbell’s standard, finding that equivalent should be classified as easy, as there is only one rendition. However, it takes a lot of effort and should be regarded as difficult. Thus, the number of different renditions may not be an effective indicator of translation difficulty.
Jensen (2009) employed three indicators of translation difficulty in his study: readability indices, word frequency, and non-literalness (that is, the number of occurrences of non-literal expressions, i.e. idioms, metaphors, and metonyms). His reasoning was that “If one employs several indicators that all point in the same direction, it is reasonable to assume that they can be used as a reliable measure” (p. 76). This approach probably worked fine for his purpose. But generally speaking, what if three indicators do not point in the same direction? For instance, text A is more complex than text B in terms of readability indices and word frequency, but has fewer non-literal expressions than text B. Can we conclude that text A is more difficult to comprehend or translate than text B? No. As mentioned in Section 3, there are actually more variables at play (such as text type, content and subject matter). Another problem with his approach is that word frequency is a factor that most readability indices take into account.
4.2.1 Grading translations
Grading translations is one way to identify translation problems. There are two basic approaches to grading in language testing: holistic and analytic (e.g., Hughes 2003). The holistic method is based on the idea that the whole is greater than the sum of its parts. Graders are usually required to provide a single grade for a translation and they have to combine all the prominent features of a translation to arrive at an overall judgment of its quality. The analytic method entails that graders assign scores for different components or characteristics of the task and then add up these scores to obtain an overall score (Sullivan 2009). In the field of translation, the analytic rubric is usually an error classification scheme. An error, by severity, can be a major one or a minor one, so a weight in the
form of a numerical value can be assigned to each error. Currently there are quite a few translation error classification schemes, e.g., SAE J2450, BlackJack, Canadian Language Quality Measurement System (Sical), ATA framework for standardized error marking, LISA (Localization Industry Standards Association) QA Model, MeLLANGE, and others (see, e.g., Dunne 2009). Take SAE J2450 (SAE 2001), originally designed for the automotive industry, as an example. Table 1 displays its error categories and points to be deducted.
Table 1
SAE J2450 Translation Quality Metric
Error category | Weight: Serious | Weight: Minor |
Wrong term | 5 | 2 |
Syntactic error | 4 | 2 |
Omission | 4 | 2 |
Word structure or agreement error | 4 | 2 |
Misspelling | 3 | 1 |
Punctuation error | 2 | 1 |
Miscellaneous error | 3 | 1 |
For the purpose of identifying translation problems, the analytic method is obviously more suitable. It should be mentioned that measuring translation quality is like judging a beauty pageant, it is an inherently subjective process and relies on human judgment. Even if it were an objective process and all translation errors were mechanical (e.g., capitalization, punctuation, single word errors), graders still would not assign the same score to the same translation. The real issue is how to minimize bias and increase the reliability and validity of the grading methods.
There are many types of grader (or rater) bias, such as central tendency (i.e., assigning scores primarily around the scale midpoint), fatigue, handwriting, leniency/severity, skimming (i.e., not reading the whole response), trait (i.e., focusing on one aspect), and others (Johnson et al. 2009:211). These biases need to be minimized via developing scoring rubrics, grader training, identifying benchmark responses that provide concrete examples of each proficiency level or exemplify each point of the scoring rubric, and other measures (Johnson et al. 2009; Linn 2006).
To facilitate grading and analysis, a software program called TransGrading (see www.transgrading.com) was developed by the author and a collaborator. It allows the user to use analytical and/or holistic grading methods; the error category list and holistic category list are customizable; it can calculate error deduction points and total scores, and export errors, error deduction points, and total scores to Microsoft Excel for further analysis.
4.2.2 Analyzing verbal protocols
The theory that verbal protocols can be used to elicit data on cognitive processes was proposed by Ericsson and Simon (1980, 1993), and they have provided substantial empirical support for it. Ericsson and Simon hold that participants can generate verbalizations, subordinated to task-driven cognitive processes, without changing the sequence of their thoughts (1993:xxxii). Ever since, analyzing think-aloud protocols (TAP) has become one of the core research methods in expertise studies (e.g., Ericsson 2006) and problem solving (e.g., Robertson 2001). In this kind of research, participants are requested to speak out their thoughts while performing a task (e.g., translating a text). This way, verbal reports provide explicit information about the knowledge and information heeded in solving a problem and from the verbal reports researchers can infer the processes (Simon and Kaplan 1989:23). For instance, if a participant thinks aloud that “The word puzzles me” or “What does this mean?”, the researcher would know that this translator encounters a problem.
Analyzing think-aloud protocols has been a major research method in translation process research since the 1980s (see Jääskeläinen 2002). In recent years, there have been some controversies as to the suitability of verbal protocol analysis for translation process research in terms of its validity and reliability. Although empirical studies are needed to solve some of the doubts and questions, to date no study has falsified this method for translation process research (see Sun 2011).
4.2.3 Recording and analyzing translation behavior
Verbal protocols are used to look into thoughts and their sequences providing verbal data. By comparison, recording methods are used to record overt behavior precisely. Their data often can be transformed into numbers and used in correlational analysis. The oft-used recording methods in translation research include keystroke logging, screen recording, and eye tracking.
A keystroke logging software tool (e.g., Translog, ScriptLog, Inputlog) records all the keyboard activity and generates a lot of recorded data consisting of information concerning pausing (where and when pauses occurred, and for how long) and the history of all keyboard actions and cursor movements; it is used to study pause location, pausing in relation to planning and discourse production, and revision behavior (see Sullivan and Lindgren 2006). If a participant pauses for a relatively long time during translation, the researcher would know where this translator has a problem (e.g., Jensen 2009). One characteristic of keystroke logging is that only the writing process involved in translating is recorded.
A keystroke logger can only record keyboard actions and cursor movements within its interface. To track the translator’s behavior outside the keystroke logger (e.g., consulting an electronic or online dictionary), screen recording is often used. Such a tool (e.g., CamStudio, Camtasia) can record all screen and audio activity on a computer and create AVI video files. Eye tracking can measure eye movements including the number of fixations, fixation durations, attentional switching, and scanpath similarity (see Duchowski 2007).
The fundamental assumption in using translation behavior data is that there is a correlation between outside behavior and cognitive processing. In most cases, however, when only one method is used, one can only speculate about the cognitive processes that a translator is engaged in (Jakobsen 2011:37). For instance, if a participant’s mind wanders during the translation process, pause data produced by keystroke logging will be misleading. In order to maximize the chance of reconstructing the translation cognitive processes, researchers tend to use multiple methods.
As verbal protocol analysis and recording and analyzing translation behavior are usually time-consuming and labor-intensive, they are suitable for studies involving a few participants and/or relatively short texts. For instance, transcribing an hour of speech can take over ten hours.
4.3.Measuring Mental Workload
Section 4.2 discussed how to locate and diagnose difficulty ‘points’ in a translation. This section addresses the question how to measure the global difficulty level of a translation for a translator.
There are a large number of techniques for measuring mental workload and they can be classified into three major categories: 1) subjective measures, 2) performance measures, and 3) physiological measures.
4.3.1 Subjective measures
Subjective measures typically involve having participants judge and report their own experience of the workload imposed by performing a specific task. The rationale for using subjective measures is that increased capacity expenditure will be associated with subjective feelings of effort or exertion that can be reported accurately by the participant unless the workload is beyond what the participant can handle (O’Donnell and Eggemeier 1986:7).
Subjective measures have been very frequently used to evaluate mental workload mainly because of their high face validity and easy implementation (Vidulich 1988), and the most commonly used subjective measure is the rating scale. Among the subjective rating scales designated specifically for assessing workload, the most frequently employed and evaluated with respect to their reliability, validity and sensitivity to change in workload are the NASA task load index (TLX), the subjective workload assessment technique (SWAT), and the Cooper–Harper scale (MCH) (Wilson and Eggemeier 2006).
Based on 16 experiments with different kinds of tasks, Hart and Staveland (1988) developed the NASA-TLX (task load index), which includes six workload-related subscales, as follows:
- Mental demand: How much mental and perceptual activity was required (e.g., thinking, deciding, remembering, searching, etc.)?
- Physical demand: How much physical activity was required (e.g., pushing, pulling, etc.)?
- Temporal demand: How much time pressure did you feel due to the rate or pace at which the tasks or task elements occurred?
- Effort: How hard did you have to work (mentally and physically) to accomplish your level of performance?
- Performance: How successful do you think you were in accomplishing the goals of the task set by the experimenter (or yourself)?
- Frustration level: How insecure, discouraged, stressed and annoyed versus secure, gratified, content, relaxed and complacent did you feel during the task?
Each subscale is presented as a line divided into 20 equal intervals anchored by bipolar descriptors (e.g., low/high, good/poor), as shown in Figure 2.
Ever since its introduction, NASA-TLX has been subjected to a number of independent evaluations with respect to its reliability, validity, sensitivity, and utility, and is now “being used as a benchmark against which the efficacy of other measures, theories, or models [of workload measurement] are judged” (Hart 2006:907). It has been adopted to evaluate the workload of tasks with focus on manual control, perception, short-term memory, cognitive processing, or parallel and serial dual-tasks.

Figure 2
A NASA-TLX subscale
In the field of cognitive load theory, a well-known and extensively used subjective rating scale is a 9-point Likert scale first used by Paas (1992). This scale ranges from “very, very low mental effort” (1) to “very, very high mental effort” (9). Later studies show that this simple subjective rating scale has been shown to be a very “sensitive measure available to differentiate the cognitive load imposed by different instructional procedures” (Sweller et al. 2011:74).
4.3.2 Performance measures
Performance measures derive an index of workload from some aspect of the participant’s behavior or activity. It is generally assumed that low to moderate levels of workload or information processing demand are associated with acceptable levels of operator performance, while high levels of workload are associated with degraded levels of operator performance (Wilson and Eggemeier 2006:814). That is, task underload may lead to artificially enhanced performance, whereas task overload may result in a ‘floor effect’ (O’Donnell and Eggemeier 1986). Thus, the relationship between workload and performance is not linear. This has been realized by researchers in the field of human factors and ergonomics since the 1970s. In an experiment utilizing functional nearinfrared spectroscopy (fNIRs) to examine the relationship between mental workload, level of expertise, and task performance. Bunce et al. (2011) found that as the level of task load in a video game moved from moderate to high, the four participants with high practice performed better, while the four novices’ performance dropped precipitously.
Two commonly used workload indicators are speed (i.e., time-on-task) and accuracy (i.e., number of errors). They have been proved to show sensitivity to workload manipulations (see O’Donnell and Eggemeier 1986:21).
4.3.3 Physiological measures
Physiological measures detect changes in the participant’s body for inferring the level of workload related to a task. There are various classification methods for them (e.g., Tsang 2006; Vice et al. 2007). For instance, O’Donnell and Eggemeier (1986) divide physiological measures into four categories, as follows:
- measures of brain function: e.g., electroencephalogram (EEG);
- measure of eye function: e.g., corneal reflex, electrooculogram (EOG); and pupil response;
- measures of cardiac function: e.g., electrocardiogram (EKG), blood pressure, heart rate, and oxygen concentration;
- measures of muscle function: e.g., electromyogram (EMG).
Compared with subjective and performance measures, physiological measures are typically “online, covert, and continuous”, and “can be linked in time to the expected occurrence of the psychological state or process being indexed, thereby providing simultaneous evidence of the strength or operation of the state or process” (Blascovich 2004:881). However, they are affected by environmental influences and the participant’s physical state, and compared with those reflective of bodily functions, the physiological signals that are indicative of mental demands could be small (Tsang 2006). When collecting data using physiological measures, researchers need to consider such factors as invasiveness, cost, sensitivity, validity, practicality, and reliability (Vice et al. 2007).
Each of the three kinds of techniques for measuring mental workload discussed in this section can provide valuable information and they have different strengths and weaknesses. Because mental workload is multidimensional and real-world tasks may involve more than one component, researchers usually use multiple workload measures in order to obtain a more complete picture of the mental workload (Tsang 2006).
Sometimes, conflicting results from multiple measures arise. For instance, several studies in the field of cognitive load theory found a cognitive load difference based on subjective measures but no group treatment effect on performance measures (see Sweller et al. 2011:75). In this case, we can follow Jex’s (1988:14) suggestion that “the fundamental measure, against which all objective measures must be calibrated, is the individual’s subjective workload evaluation in each task”.
5. CONCLUSION AND QUESTIONS FOR FUTURE RESEARCH
This article has explored sources of translation difficulty and ways for measuring them in operational terms, drawing on theories and findings in many other research fields (such as reading, writing, cognitive psychology, human factors) as well as in translation studies. Thereby it may help create a solid foundation for this line of inquiry.
So far, there have been few empirical studies in this field despite the thriving of translation process research. Questions that require empirical investigation
include, among others: 1) which measures from the aforementioned subjective, performance, and physiological measures are reliable, sensitive, and unobtrusive in measuring translation difficulty; 2) which variables (e.g., translation quality scores, time on task) can be used to measure or represent translation difficulty; 3) whether readability formulas can be used to predict a text’s level of translation difficulty; 4) whether environmental variables (e.g., task criticality, time pressure, availability of reference materials, equipment used) would influence the translator’s perception of a text’s level of translation difficulty; 5) whether novice and professional translators rank the difficulty levels of a group of translation tasks in the same way; 6) whether a senior translation major would assign the same difficulty score to a text in her rating as she did three years ago.
Research in translation difficulty can expand the focus of translation process research, and contribute greatly to our understanding of translation process in terms of relationships between text characteristics, translator behaviors, and translation quality. It will also benefit translation pedagogy and accreditation. Our vision is that a working model for assessing translation difficulty will be proposed and a computer program for measuring translation difficulty between a specific language pair will become available in the near future.
References
Alderson, J. C. 2000. Assessing Reading. Cambridge: Cambridge University Press.
Anagnostou, N. K., & Weir, G. R. S. 2007. From Corpus-based Collocation Frequencies to Readability Measure. In: Weir G. R. S. & Ozasa, T. (eds) Texts, Textbooks and Readability. Glasgow: University of Strathclyde Publishing. 34–48.
Baker, M. 1992. In Other Words: a Coursebook on Translation. London; New York: Routledge.
Baker, M. 2011. In Other Words: a Coursebook on Translation (2nd ed.). New York: Routledge.
Biber, D. 1988. Variation Across Speech and Writing. New York: Cambridge University Press.
Biber, D. 1989. A Typology of English Texts. Linguistics Vol. 27. No. 1. 3–44.
Blascovich, J. 2004. Psychophysiological Measures. In: Lewis-Beck, M.S. Bryman, A. & Liao, T.F. (eds) The Sage Encyclopedia of Social Science Research Methods. Thousand Oaks, Calif.: Sage. Vol. 1. 881–883.
Bradshaw, J. L. 1968. Load and Pupillary Changes in Continuous Processing Tasks. British Journal of Psychology Vol. 59. No. 3. 265–271.
Bunce, S. C., Izzetoglu, K., Ayaz, H., Shewokis, P., Izzetoglu, M., Pourrezaei, K., et al. 2011. Implementation of fNIRS for Monitoring Levels of Expertise and Mental Workload. In: Schmorrow, D. D. & Fidopiastis, C.M. (eds) Foundations of Augmented Cognition: Directing the Future of Adaptive Systems. New York: Springer. 13–22.
Burns, M. K. 2004. Empirical Analysis of Drill Ratio Research: Refining the Instructional Level for Drill Tasks. Remedial and Special Education Vol. 25. No. 3. 167–173.
Campbell, S., & Hale, S. 1999. What Makes a Text Difficult to Translate? Refereed Proceedings of the 23rd Annual ALAA Congress. Retrieved Nov. 1, 2009, from http://www.latrobe.edu.au/alaa/proceed/camphale.html
Campbell, S. & Hale, S. 2003. Translation and Interpreting Assessment in the Context of Educational Measurement. In: Anderman, G. M. & Rogers, M. (eds) Translation Today: Trends and Perspectives. Clevedon; Buffalo, N.Y.: Multilingual Matters. 205–224.
Carver, R. P. 1975–1976. Measuring Prose Difficulty Using the Rauding Scale. Reading Research Quarterly Vol. 4. No. 11. 660–685.
Castello, E. 2008. Text Complexity and Reading Comprehension tests. Bern; New York: Peter Lang.
Chall, J. S., Bissex, G. L., Conard, S. S., & Harris-Sharples, S. H. 1996. Qualitative Assessment of Text Difficulty: a Practical Guide for Teachers and Writers. Cambridge, Mass.: Brookline Books.
Chall, J. S., & Dale, E. 1995. Readability Revisited: The New Dale-Chall Readability Formula. Cambridge, Mass.: Brookline Books.
Chi, M. T. H. 2006. Two Approaches to the Study of Experts’ Characteristics. In: Ericsson, K.A., Charness, N., Feltovich, P. J. & Hoffman, R. R. (eds) The Cambridge Handbook of Expertise and Expert Performance. Cambridge: Cambridge University Press. 21–30.
Dahl, Ö. 2004. The Growth and Maintenance of Linguistic Complexity. Amsterdam; Philadelphia: John Benjamins.
Dragsted, B. 2004. Segmentation in Translation and Translation Memory Systems: An Empirical Investigation of Cognitive Segmentation and Effects of Integrating a TM-System into the Translation Process. Unpublished PhD dissertation, Copenhagen Business School.
DuBay, W. H. 2004. The Principles of Readability. Retrieved November 1, 2009, from http://www.impact-information.com/impactinfo/readability02.pdf
Duchowski, A. T. 2007. Eye Tracking Methodology: Theory and Practice (2nd ed.). London: Springer.
Dunne, K. 2009. Assessing Software Localization: For a Valid Approach. In: Angelelli, C. & Jacobson, H. E. (eds) Testing and Assessment in Translation and Interpreting Studies: A Call for Dialogue Between Research and Practice. Amsterdam; Philadelphia: John Benjamins. 185–222.
Englund Dimitrova, B. 2005. Expertise and Explicitation in the Translation Process. Amsterdam: John Benjamins.
Ericsson, K. A. 2006. Protocol Analysis and Expert Thought: Concurrent Verbalizations of Thinking during Experts’ Performance on Representative Tasks. In: Ericsson, K. A., Charness, N., Feltovich, P. J. & Hoffman, R. R. (eds) The Cambridge Handbook of Expertise and Expert Performance. Cambridge: Cambridge University Press. 223–241.
Ericsson, K. A. & Simon, H. A. 1980. Verbal Reports as Data. Psychological Review Vol. 87. No.3. 215–251.
Ericsson, K. A. & Simon, H. A. 1993. Protocol Analysis: Verbal Reports as data (Rev. ed.).Cambridge, Mass.: MIT Press.
Flesch, R. 1948. A New Readability Yardstick. Journal of Applied Psychology Vol. 32. No. 3. 221–233.
Fraser, J. 1996. The Translator Investigated: Learning From Translation Process Analysis. The Translator Vol. 2. No. 1. 65–79.
Fry, E. B. 1989. Reading Formulas: Maligned but Valid. Journal of Reading Vol. 32. No. 4. 292– 297.
Gickling, E. & Rosenfield, S. 1995. Best Practices in Curriculum-based Assessment. In: Thomas, A. & Grimes, J. (eds) Best Practices in School Psychology. Washington, DC: National Association of School Psychologists. 3rd ed., 587–595.
Gopher, D. & Donchin, E. 1986. Workload: An Examination of the Concept. In: Boff, K. R., Kaufman, L. & Thomas, J. P. (eds) Handbook of Perception and Human Performance, Vol. II: Cognitive Processes and Performance. New York: Wiley. 41/41–41/49.
Graesser, A. C., McNamara, D. S. & Louwerse, M. M. 2011. Methods of Automated Text Analysis. In: Kamil, M.L., Pearson, P.D., Moje, E. D. & Afflerbach, P.P. (eds) Handbook of Reading Research, Volume IV. New York: Routledge. 34–53.
Gray, W. S. & Leary, B. E. 1935. What Makes a Book Readable. Chicago, Ill.,: The University of Chicago Press.
Hale, S. & Campbell, S. 2002. The Interaction Between Text Difficulty and Translation Accuracy. Babel Vol. 48. No. 1. 14–33.
Hart, S. G. 2006. NASA-Task Load Index (NASA-TLX); 20 Years Later. Proceedings of the Human Factors and Ergonomics Society 50th Annual Meeting. Santa Monica: Human Factors and Ergonomics Society. 904–908.
Hart, S. G. & Staveland, L. E. 1988. Development of NASA-TLX (Task Load Index): Results of Empirical and Theoretical Research. In: Hancock, P. A. & Meshkati, N. (eds) Human Mental Workload. Amsterdam: New York: North-Holland. 139–183.
Hatim, B. & Mason, I. 1997. The Translator as Communicator. London: Routledge. Hughes, A. 2003. Testing for Language Teachers (2nd ed.). Cambridge; New York: Cambridge University Press.
Jääskeläinen, R. 1999. Tapping the Process: An Explorative Study of the Cognitive and Affective Factors Involved in Translating. Joensuu: University of Joensuu Publications in the Humanities. No 22.
Jääskeläinen, R. 2002. Think-aloud Protocol Studies into Translation: An Annotated Bibliography. Target Vol. 14. No.1. 107–136.
Jakobsen, A. L. 2011. Tracking Translators’ Keystrokes and Eye Movements with Translog. In C. Alvstad, A. Hild & E. Tiselius (eds) Methods and Strategies of Process Research: Integrative Approaches in Translation Studies. Amsterdam; Philadelphia: John Benjamins. 37–55.
Jensen, K. T. 2009. Indicators of Text Complexity. Copenhagen Studies in Language Vol. 37.61–80.
Jex, H. R. 1988. Measuring Mental Workload: Problems, Progress, and Promises. In:. Hancock, P.A. & Meshkati, N. (eds) Human Mental Workload. Amsterdam; New York: North-Holland. 5–38.
Johnson, R. L., Penny, J. A. & Gordon, B. 2009. Assessing Performance: Designing, Scoring, and Validating Performance Tasks. New York: The Guilford Press. Kalyuga, S. 2009. Managing Cognitive Load in Adaptive Multimedia Learning. Hershey, PA: Information Science Reference.
Kamil, M. L., Pearson, P. D., Moje, E. B. & Afflerbach, P. P. (eds) 2011. Handbook of Reading Research, Volume IV. New York: Routledge.
Karwowski, W. (ed.) 2006. International Encyclopedia of Ergonomics and Human Factors (2nd ed.). Boca Raton, FL: CRC/Taylor & Francis.
Kenny, D. 2009. Equivalence. In: Baker, M. & Saldanha, G. (eds) Routledge Encyclopedia of Translation Studies. London; New York: Routledge. 2nd ed., 96–99. Klare, G. R. 1974–1975. Assessing Readability. Reading Research Quarterly Vol. 10. No. 1.62–102.
Klare, G. R. 1984. Readability. In: Pearson, P. D. & Barr, R. (eds) Handbook of Reading Research. New York: Longman. 681–744.
Krings, H. P. 2001. Repairing Texts: Empirical Investigations of Machine Translation Postediting Processes (Koby, G., Shreve, G., Mischerikow, K. & Litzer, S. Trans.). Kent, Ohio: Kent State University Press.
Lee, D. Y. 2001. Genres, Registers, Text Types, Domains, and Styles: Clarifying the Concepts and Navigating a Path Through the BNC Jungle. Language Learning and Technology Vol. 5. No. 3. 37–72.
Linn, R. L. 2006. The Standards for Educational and Psychological Testing: Guidance in Test Development In: Downing, S. M. & Haladyna, T. M. (eds) Handbook of Test Development. Mahwah, N.J.: L. Erlbaum. 27–38.
Lohman, D. F. & Lakin, J. M. 2011. Intelligence and Reasoning. In: Ternberg, R. J. & Kaufman,
S. B. (eds) The Cambridge Handbook of Intelligence. Cambridge; New York: Cambridge University Press. 419–441.
Lonsdale, A. B. 2009. Directionality. In: Baker, M. & Saldanha, G. (eds) Routledge Encyclopedia of Translation Studies. London; New York: Routledge. 2nd ed., 84–88.
Lörscher, W. 1986. Linguistic Aspects of Translation Processes: Towards an Analysis of Translation Performance. In: House, J. & Blum-Kulka, S. (eds) Interlingual and Intercultural Communication: Discourse and Cognition in Translation and Second Language Acquisition Studies. Tübingen: Gunter Narr. 243–262.
Meshkati, N. 1988. Toward Development of a Cohesive Model of Workload. In: Hancock, P. A. & Meshkati, N. (eds) Human Mental Workload. Amsterdam; New York: North-Holland. 305–314.
Moessner, L. 2001. Genre, Text Type, Style, Register: A Terminological Maze? European Journal of English Studies Vol. 5. No. 2. 131–138.
Moray, N. 1979. Mental Workload: Its Theory and Measurement. New York: Plenum Press. Neubert, A. 2000. Competence in Language, in Languages, and in Translation. In: Schäffner, C. & Adab, B. (eds) Developing Translation Competence. Amsterdam; Philadelphia: John Benjamins. 3–18.
Nord, C. 1991. Text Analysis in Translation: Theory, Methodology, and Didactic Application of a Model for Translation-oriented Text Analysis. Amsterdam: Rodopi.
Nord, C. 2005. Text Analysis in Translation: Theory, Methodology, and Didactic Application of a Model for Translation-oriented Text Analysis (2nd ed.). Amsterdam: Rodopi.
O’Donnell, R. D. & Eggemeier, F. T. 1986. Workload Assessment Methodology. In: Boff, K. R., Kaufman, L. & Thomas, J. P. (eds) Handbook of Perception and Human Performance, Vol. II: Cognitive Processes and Performance. New York: Wiley. 42/41–42/49.
Ott, L. & Longnecker, M. 2010. An Introduction to Statistical Methods and Data Analysis (6th ed.). Australia; United States: Brooks/Cole Cengage Learning. Paas, F. G. 1992. Training Strategies for Attaining Transfer of Problem-solving Skill in Statistics: A Cognitive-load Approach. Journal of Educational Psychology Vol. 84. No. 4. 429–434.
PACTE. 2003. Building a Translation Competence Model. In: Alves, F. (ed.) Triangulating Translation: Perspectives in Process Oriented Research. Amsterdam: John Benjamins Pub. 43–66.
Plass, J. L., Moreno, R. & Brünken, R. 2010. Introduction. In: Plass, J. L., Moreno, R. & Brünken, R. (eds) Cognitive Load Theory. Cambridge; New York: Cambridge University Press. 1–6. Pym, A. 2003. Redefining Translation Competence in an Electronic Age: In Defence of a Minimalist Approach. Meta Vol. 48. No. 4. 481–497.
Pym, A. 2010. Exploring Translation Theories. London/New York: Routledge. RAND. 2002. Reading for understanding: toward a research and development program in reading comprehension Available from http://www.rand.org/pubs/monograph_reports/2005/ MR1465.pdf
Rayner, K. & Pollatsek, A. 1989. The Psychology of Reading. Hillsdale, N.J.: Lawrence Erlbaum. Robertson, S. I. 2001. Problem Solving. Philadelphia: Psychology Press.
Robinson, P. (ed.) 2011. Second Language Task Complexity: Researching the Cognition Hypothesis of Language Learning and Performance. Amsterdam; Philadelphia: John Benjamins.
SAE. 2001. Translation Quality Metric [J2450]. Warrendale, PA: SAE.
Sammer, G. 2006. Workload and Electro-encephalography Dynamics. In: Karwowski, W. (ed.) International Encyclopedia of Ergonomics and Human Factors. Boca Raton, FL: CRC/Taylor & Francis. 2nd ed., Vol. 1, 561–564.
Shreve, G. M. 2002. Knowing Translation: Cognitive and Experiential Aspects of Translation Expertise from the Perspective of Expertise Studies. In: Riccardi, A. (ed.) Translation Studies: Perspectives on an Emerging Discipline. Cambridge/New York: Cambridge University Press. 150–171.
Shreve, G. M., Danks, J. H. & Lacruz, I. 2004. Cognitive Processes in Translation: Research Summary for the Center for the Advanced Study of Language, University of Maryland.
Simon, H. A., & Kaplan, C. 1989. Foundations of Cognitive Science. In: Posner, M. I. (ed.) Foundations of Cognitive Science. Cambridge, Mass.: MIT Press. 1–47.
Sin, I. Y. F. 2011. Insights from book translations on the international diffusion of knowledge. Unpublished dissertation, Stanford University.
Snell-Hornby, M. 1988. Translation Studies: An Integrated Approach. Amsterdam; Philadelphia: John Benjamins.
Sullivan, K. P. H. & Lindgren, E. (eds) 2006. Computer Keystroke Logging and Writing: Methods and applications. Amsterdam; London: Elsevier.
Sullivan, L. E. (ed.) 2009. The SAGE Glossary of the Social and Behavioral Sciences. London; Thousand Oaks, CA: SAGE.
Sun, S. 2011. Think-Aloud-Based Translation Process Research: Some Methodological Considerations. Meta Vol. 56. No. 4. 928–951.
Sweller, J. 1988. Cognitive Load during Problem Solving: Effects on Learning. Cognitive Science Vol. 12. No. 2. 257–285.
Sweller, J., Ayres, P. L. & Kalyuga, S. 2011. Cognitive Load Theory. New York; London: Springer.
Taylor, W. L. 1953. “Cloze procedure”: A New Tool for Measuring Readability. Journalism Quarterly Vol. 30. 415–433.
Tirkkonen-Condit, S. 1987. Think-aloud protocols in the study of the translation process. In: Nyyssönen, H., Kataja, R. & Komulainen, V. (eds) CDEF 86: Papers from the conference of departments of English in Finland. Oulu: University of Oulu. 39–49. Trosborg, A. (ed.) 1997. Text Typology and Translation. Amsterdam: John Benjamins. Tsang, P. S. 2006. Mental Workload. In: Karwowski, W. (ed.) International Encyclopedia of Ergonomics and Human Factors. Boca Raton, FL: CRC/Taylor & Francis. 2nd ed., Vol. 1, 809–813.
Urdan, T. C. 2010. Statistics in Plain English (3rd ed.). New York: Routledge.
Usó-Juan, E. 2006. The Compensatory Nature of Discipline-Related Knowledge and EnglishLanguage Proficiency in Reading English for Academic Purposes. The Modern Language Journal Vol. 90. No. 1. 210–227.
Vice, J. M., Lathan, C., Lockerd, A. D. & Hitt, J. M. 2007. Simulation Fidelity Design Informed by Physiologically-based Measurement Tools. In: Schmorrow, D. D. & Reeves, L. M. (eds) Foundations of augmented cognition: third international conference, FAC 2007, held as part of HCI international 2007, Beijing, China, July 22–27, 2007 proceedings. Berlin/New York: Springer. 186–194.
Vidulich, M. A. 1988. The Cognitive Psychology of Subjective Mental Workload. In: Hancock, P. A. & Meshkati, N. (eds) Human Mental Workload. Amsterdam/New York: North-Holland. 219–229.
Wilson, G. F. & Eggemeier, F. T. 2006. Mental Workload Measurement. In: Karwowski, W. (ed.) International Encyclopedia of Ergonomics and Human Factors. Boca Raton, FL: CRC/Taylor & Francis. 2nd ed., Vol. 1. 814–817.
Wilss, W. 1976. Perspectives and Limitations of a Didactic Framework for the Teaching of Translation. In: Brislin, R. W. (ed.) Translation: Applications and Research. New York: Gardner Press. 117–137.
Wilss, W. 1982. The Science of Translation: Problems and Methods. Tübingen: G. Narr. Zipf, G. K. 1935. The Psycho-biology of Language: An Introduction to Dynamic Philology. Boston: Houghton Mifflin.